Feature
Can pharma overcome generative AI’s bias problem?
While the use of generative AI to optimise the practitioner-to-patient relationship grows, ethical questions over bias thwart pharma’s reliance on the tech. Natasha Spencer-Jolliffe reports.
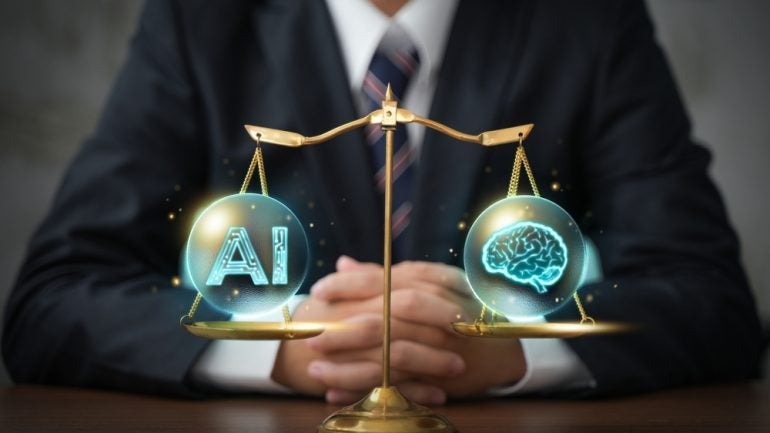
Generative AI has huge potential for healthcare, but is challenged by bias. Credit: Shutterstock/Sansoen Saengsakaorat
Generative artificial intelligence (AI) or gen AI refers to algorithms that can produce new content such as codes, text, images and simulations. Tools such as ChatGPT and chatbots provide an efficient, personalised and easier-to-manage method to inform patients about trials and treatments.
“Within a decade, the biopharma industry may use AI to speed product development and create dozens of completely new therapies,” Florian Schnappauf, vice president of Enterprise Commercial Strategy, Veeva Europe, tells Pharmaceutical Technology.“ AI-powered insights can support more relevant conversations about new specialised treatments, helping healthcare professionals effectively deliver care to the right patients,” Schnappauf adds.
However, the technology is new. “In an industry marked by stringent regulations, the adoption of gen AI is still in its nascent stages,” says Nutan B, VP of consulting at data science company Gramener. Despite its infancy, however, gen AI holds considerable potential to transform pharmaceutical research and development (R&D), clinical trials, and patient engagement.
Still, with these new technological capabilities come increasing concerns around ethics. These have to do with the ability of gen AI applications to identify biases related to race, gender, and age and the critical need to eliminate them. Questions on how inherent biases can negatively affect clinical trials and drug development prompt caution, and posit if better AI algorithmic training can avoid these pitfalls.
Raising questions on ethics
The ethics of AI is an active area of debate in pharma, with scientists fielding ethical questions on gen AI. “In highly regulated industries, adopting analytics as a capability has always been challenging,” says B. Analytics rely on probabilities, which differ from the deterministic approaches these industries are accustomed to, creating hurdles that gen AI tools only complicate.
“Unaddressed biases can lead to significant disparities in disease diagnosis or drug recommendations,” says B. In healthcare settings, it is crucial to establish when and how patients are informed about the role of technology in their treatment, clinical trials, or diagnostic recommendations.
In June 2023, UNESCO and the European Commission signed an agreement to pursue the global implementation of the ethics of AI. Adopted in November 2021 by 193 Member States, it strives to advance accountability and the rule of law, including concrete policy items calling for better data governance, inclusivity and gender equality.
Organisations like the Partnership on Ethical AI are actively involved in finding ways to mitigate the impact of such biases. However, it is up to individual organisations and teams working with gen AI to incorporate strategies for ensuring fairness into their solutions.
Legal precedents in intellectual property, copyright, and informed consent are still underway. Data privacy and security are essential dimensions to consider, particularly in the research community, and solutions are emerging from a technological and infrastructural standpoint.
Potential to identify biases?
Generative models, like any other tool, cannot inherently detect biases independently. “Despite the name, AI is not intelligent in a human sense and does not understand what it says, let alone [understand] bias,” Daniel Schlagwein, PhD, Associate Professor at The University of Sydney and editor-in-chief (co) of the Journal of Information Technology, tells Pharmaceutical Technology. Gen AI primarily learns from the data it is trained on. “If the data itself contains biases, there’s a high likelihood that the AI will replicate these biases in its outputs,” says B.
Researchers, scientists and developers need to create processes and solutions to mitigate these biases. Testing evaluation techniques to identify biases, specific solution designs and defining endpoints and procedures are applicable methods. Multiple datasets can be designed to evaluate fairness across attributes like race, gender, and age.
“These processes could be time-consuming or dampen the excitement of implementing generative applications and add some overheads. Though they are highly crucial for gen AI to be sustainably used in the future,” says B.
Biases in drug trials and development
An August 2023 research study found that bias is a major concern in generative AI, with further research required to examine these biases that can be attributable to training datasets and processes.
“Inherent biases pose a significant threat to the integrity of clinical trials and drug development, potentially resulting in severe consequences if left unaddressed,” says B. The root of this issue lies in skewed data, she continues, particularly concerning the underrepresentation of specific demographics in datasets related to conditions such as cancer and heart disease. This bias could be passed on to gen AI and its applications without careful management.
Multiple steps in a clinical trial are impacted by these biases, starting with patient recruitment, protocol design assessment, and data analysis. The lack of diversity in trial participants can introduce biases in comprehending how drugs affect different ethnic groups, genders, and demographics, which B says can “ultimately undermine the applicability of the trial’s results”.
Implementing data safety monitoring boards and mandating the publication of all clinical trial results can contribute significantly to creating more equitable and unbiased outcomes, prioritising patient wellbeing.
Training AI algorithms
Open source, which refers to publicising data and code, enables AI algorithms to become better trained to avoid these pitfalls. “Completing the training data publicly—even putting it on public blockchains— and the programming code open source should be fundamental,” says Schlagwein.
OpenAI’s advanced system, GPT 4, a large multimodal model, is trained on a trillion parameters. “Fundamentally, retraining these algorithms is becoming almost impossible for individual healthcare and pharmaceutical organisations,” says B.
Fine-tuning techniques that leverage representative training data prioritising diversity in race, gender, age, and geography are available and can correct the biases to a certain extent. Solution approaches like data augmentation, adversarial training and designing human-in-the-loop approaches to get expert feedback can also support in addressing bias.
“Explainability and interpretability play crucial parts in both the technology adoption and in addressing the bias problem,” says B.
Eradicating bias requires more than AI
Overarching frameworks from regulatory bodies like the US Food and Drug Administration (FDA) or World Health Organisation (WHO) and organisations themselves can serve as a roadmap for developing these algorithms, prioritising ethical principles, fairness and transparency.
Addressing ethical questions with gen AI requires multiple layers and actions. Firstly, researchers and scientists need to build AI systems with inherent ethical considerations, emphasising fairness, transparency, and accountability. Next, regulators or regulatory mechanisms must establish clear instructions for responsible AI deployment. Lastly, defining the areas for AI use is crucial. “In principle, [this] is the big ticket item – and this is still not thoroughly debated, let alone resolved,” says Schlagwein.