Insight
What can life sciences firms really achieve with AI?
Artificial intelligence has been one of the most hyped technologies of the past decade. It is used by organisations in a variety of sectors in a multitude of ways, and for an industry such as life sciences the potential for AI to transform is rich. But what is achievable, in both the short term and long term? PwC’s Peter Brandstetter shares his expertise.
Every technology goes through different stages of hype when it first emerges. Industry analyst group Gartner has even coined the term ‘Hype Cycle’ to refer to this evolution, used by organisations to help discern the hype from what's commercially viable. A Hype Cycle provides a graphical overview of the maturity of a particular technology and how it is being used to solve real business issues.
That’s exactly where artificial intelligence (AI) finds itself now. The potential it has to transform many elements of life sciences is vast and we are near the inflection point when AI (and related technologies such as IoT, analytics and big data) is moving beyond the hype into reality.
I spoke at the AMPLEXOR BE THE EXPERT Conference in June and mentioned some IBM research that had a statistic revealing that between 20% and 30% of pharmaceutical companies have already started with projects using AI technology. And all through the conference there were other speakers talking about using AI, how you can use it now and in the future and real world examples of organisations doing so.
The AI potential
In fact, it can be easy to get carried away with all of the potential opportunities AI, including machine learning, natural language processing and cognitive image processing, offer to life sciences firms. And it’s with good reason. As in the broader medical field, AI offers to cut to the chase and deliver new discoveries in a fraction of the time that human capabilities could match, for instance.
AI offers to cut to the chase and deliver new discoveries in a fraction of the time
This is due to the scope for mining vast vaults of data spanning case notes and academic archives from across the world, and spanning decades – aided by intensely powerful processing capacity. And intelligent systems don’t just present a chance to scour that data for interesting correlations that would be invisible to the human eye; they can also quickly hone their efforts as they learn what to look out for.
The time for AI innovation is now
Companies must have a plan for what they hope to get out of the technology. They need to ground their vision in tangible projects, and work back to a practical starting point – such as how they might optimise current systems, and rethink processes to take advantage of the best and most immediate opportunities.
What’s really interesting about AI in life sciences right now is that, that is exactly what is starting to happen. One example is a patient data box that IBM is developing in the pharmacovigilance space. It makes the whole process easier when a person with a reaction comes in, as it makes assessing it much faster – a pharmaceutical company needs to react to this within 15 days. They need to analyse what the event was, what was the cause of it, was it something that could be repeated? They need to put a lot of things together. This is known as a literature research, which is supported by what's called patient safety, a natural language processing artificial intelligence technology.
Machine learning is particularly potent when you have thousands of parameters that you need to combine
Rather than several people reading hundreds of documents, the user simply presses a button and they get the results much faster. Then the person must go and deep dive, but this AI computing at the literature research phase can be invaluable.
AI can also play a major role in generating fresh insight from existing data. One example is around one muscular skeletal disease, with data that's been around for 20 years, and has been used by many researchers and many pharma companies already. By applying machine learning, after working just three or four weeks, new insight was uncovered.
Machine learning is particularly potent when you have thousands of parameters that you need to combine and you need to look and to find patterns. Machine learning is a deep neural network and this is something that a human with traditional statistical methods cannot do as fast.
Challenges for adoption
Despite the potential it offers, AI in life sciences is not without its challenges. Life sciences is an industry in which organisations are usually not that quick to adopt new technology. It's a highly regulated industry, so every technology that is innovative also needs to prove that it's really working.
This is especially so when you use artificial intelligence, but it is also harder, as we can't use the traditional approaches where you put in A and B comes out. With AI you train the system similarly to how you would a human being, so it's not that obvious what is really coming out.
AI in life sciences is not without its challenges
Such challenges will not prevent AI from reaching its true potential in life sciences. AI is already a reality for many in the industry, while others are still in the pilot phase. But as soon as the early adopters can demonstrate strong ROI and tangible results, then the rest of the industry will follow.
Peter Brandstetter
Peter Brandstetter is a senior manager at PwC. Peter has experience across the fields of IT in life sciences industry, in particular the pharmaceutical sector. His areas of specialisation are quality management and quality assurance in manufacturing and R&D (SAP QM, LIMS, ELN), enterprise content management and R&D (clinical data management, pre-clinical, R&D Lab, R&D collaboration and project management) and computer validation.
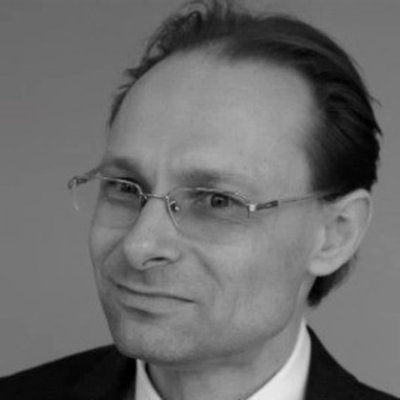
This article is based on a presentation given at AMPLEXOR’s Be The Expert training and conference in June, when the author was working for IBM Services.
Go to top