Company Insight
Sponsored by Avenga
Generative AI's leap: what LLMs bring to pharma and life sciences
Olena Domanska, Avenga’s Data Science Engineering Manager, discusses the past and the future of LLMS and their impact on pharma and biotech companies.
Main image: Generative AI can make pharma and life sciences more efficient. Credit (all images): Avenga
Generative AI has been changing various industries. Its impact on the pharmaceutical sector and life sciences is particularly noteworthy. Initially, large language models (LLMs), a subset of generative AI models, were used for text generation, but their capabilities have rapidly expanded to include complex tasks like drug discovery and development. These foundation models’ ability to work with more modalities of data, including image, video, or audio, is opening up new vistas of innovation. While more powerful LLMs like GPT-5 are on their way, companies experiment with open-access generative AI tools AI. The question is not if but how to embrace this technology.
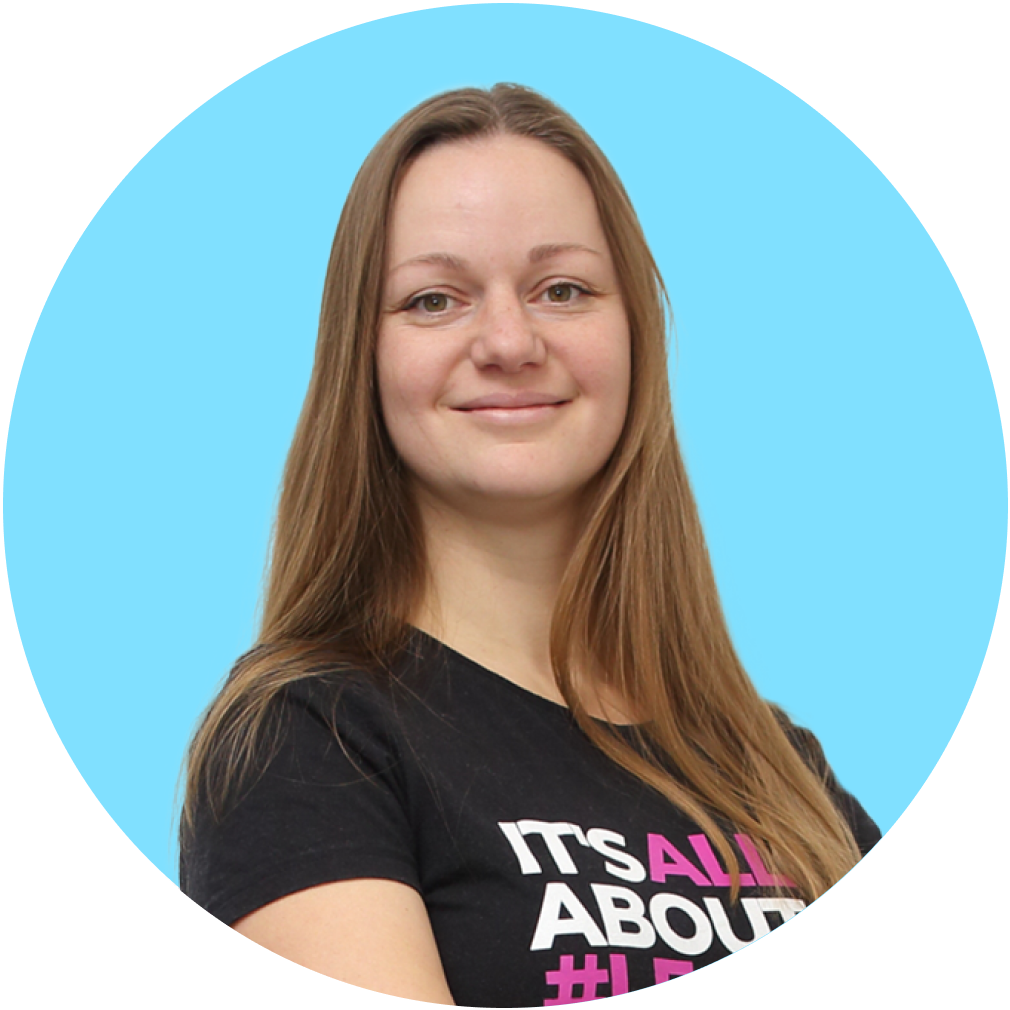
Olena Domanska, Data Science Engineering Manager
The emergence of LLMs
An LLM is a type of foundation model, specifically designed to understand and generate human language. Foundation models are AI models trained on massive amounts of data that serve as a basis for various tasks. Most modern LLMs use a transformer architecture, which is a neural network for processing sequential data like text. The transformer architecture allows the model to weigh the importance of different words in a sentence and capture long-range dependencies between words.

As one of the driving forces behind the generative AI boom, LLMs have a long history rooted in decades of research and development. These roots of LLMs trace back to the early days of AI research, with the development of basic language models in the 1950s and 60s. However, it wasn't until the rise of deep learning and neural networks in the 2010s that LLMs truly took off. The groundbreaking introduction of transformer architecture in 2017 further accelerated the development of LLMs. It made it possible for them to process longer text sequences and capture complex language patterns.
New models rising on the horizon
Some of the most well-known examples of LLMs include GPT (Generative Pre-trained Transformer) models developed by OpenAI, Claude by Anthropic, and Gemini by Google (see Fig. 1 for more). Major technology players like Microsoft, Google, and Amazon have recently launched an ‘arms race’ to make their LLMs bigger and better. This is a task that signifies a quest for more data and more sophisticated computer chips.
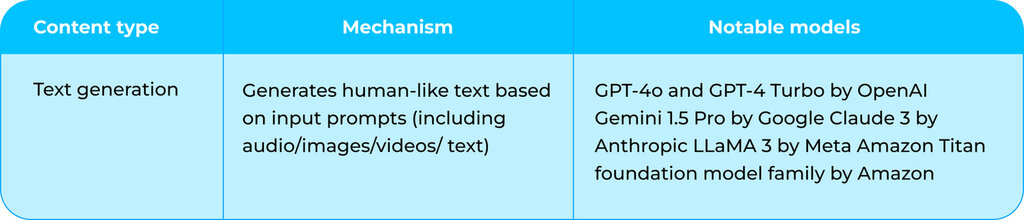
Currently, extensive investment is being poured into the development of new LLM models. This influx of resources is expected to drive the emergence of even more powerful and sophisticated models. These next-generation LLMs are likely to exhibit improved capabilities, such as the ability to perform multi-step reasoning tasks, analyze complex questions with greater precision, and potentially even exhibit a deeper understanding of nuanced language and context.
An opportunity for pharmaceutical and biotech companies
Although LLMs are nothing new to pharma and life sciences, access to them drives new ways for experiments. Here are some of the most promising use cases that LLMs now offer:
- Knowledge management and synthesis. LLMs can efficiently process and synthesize vast amounts of scientific literature, clinical trial data, and regulatory information. Their analysis can enable researchers to quickly access relevant information and gain insights that would otherwise take significantly longer.
- Drug repurposing and adverse event prediction. LLMs can sift through large datasets and pinpoint potential new uses for existing drugs (drug repurposing) and predict potential adverse events associated with new or existing drugs.
- Personalized medicine and treatment optimization. After analyzing patient data, genomic information, or medical history, LLMs can help create personalized treatment plans. In that way, intelligent patient assistants could predict treatment responses and recommend optimal dosages. Markedly, strict professional oversight would be necessary in each case.
- Clinical trial optimization. LLMs can find suitable patient populations, predict recruitment rates, and examine trial data in real-time. They can speed up clinical trials and improve patient recruitment.
- Medical writing and communication. Clear and concise medical writing that includes clinical study reports or regulatory submissions can lean on LLMs. Besides, there is an opportunity to use models for communication with patients.
- Chatbots and virtual assistants. Companies can improve patient engagement and adherence to treatment plans through chatbots and virtual assistants. They can provide personalized support and education to patients, answer questions, or offer emotional support.
The future of LLMs in pharma and life sciences is not merely increased automation or efficiency, but a paradigm shift towards augmented intelligence. LLMs will evolve into sophisticated partners, capable of deciphering intricate biological patterns or generating new hypotheses.
Multi-agent systems on the horizon
Another crucial development comes from multi-agent systems. In simple terms, a multi-agent system (MAS) is a group of LLMs that work together, each with its own specific area of expertise. These individual agents share knowledge and coordinate their actions to achieve common goals.
The potential of multi-agent LLMs in the pharmaceutical industry and life sciences is vast. For example, researchers could build an MAS where one agent specializes in analyzing vast datasets of molecular structures, another in predicting potential drug interactions, and a third in identifying optimal synthesis pathways. The united effort could level up the drug discovery process to the extent unimaginable before.
Final remarks
It seems like news about breakthroughs in generative AI hit the headlines every couple of weeks, if not days. This tempo places pressure on pharma and biotech companies to stay informed and adaptable. The key here is to understand the capabilities of LLMs and integrate them into the day-to-day work. But, and this is a big but, none of this can happen without solid rules in place that will protect patient data and make sure AI is used responsibly. Otherwise, it's like building a skyscraper on shaky ground.
Curious about the potential impact of LLMs on your company? Get in touch!