Comment
Creating a sure data footing for AI
The transformation potential of smart IT systems and process automation relies on the quality and completeness of the underlying data being drawn from across the organisation. Here, Steve Gens of Gens & Associates and Remco Munnik of Iperion Life Sciences Consultancy offer five best-practice tips for achieving and maintaining consistent enterprise-wide product data that can be trusted to drive AI-enabled innovation.
The substantial potential of emerging technologies including artificial intelligence/machine learning (AI/ML) in rapid data analysis, complexing trending and scenario analysis, and for transforming process delivery through intelligent workflow automation, has not been lost on the pharma industry.
Too often, however, organisations pursue the sharp end of innovation without first assessing and transforming the quality and reliability of the data such technologies will draw on. The steps below must be followed if organisations are to have faith in their AI-driven decisions and process improvements.
1
Assigning dedicated roles and responsibility around data quality
Having someone whose remit clearly includes maintaining the integrity and value of data is the only way to ensure any future activities drawing on these sources can be relied upon, and will stand up under regulatory scrutiny.
A 2018 study of Regulatory Information Management by Gens & Associates, which polled respondents from 72 companies internationally about their associated plans and practices, found that confidence in product registration, submission forecasting, and regulatory intelligence data quality was not high. When ‘confidence’ is low or moderate, organisations spend considerable time ‘verifying’ and remediating this information, with a direct negative impact on productivity.
Ongoing oversight over data quality is critical too, to ensure human errors do not build over time, eroding confidence in system data. Data quality sustainability should be an organisation-wide concern, necessitating a culture of quality and clear accountability for this as part of people’s roles - as appropriate.
Allocated responsibilities should ideally include:
Quality control analysis
Someone who regularly reviews the data for errors - for example sampling registration data to see how accurate and complete it is.
Data scientist
Someone who works with the data, connecting it with other sources or activities. For example, linking the company’s regulatory information management (RIM) system into clinical or ERP systems, with the aim of enabling something greater than the sum of the parts – such as ‘big picture’ analytics.
Chief data
officer
With a strategic overview across key company data sources, this person is responsible for ensuring that enterprise information assets globally - including enterprise resource planning (ERP), RIM and safety systems - have the necessary governance, standards and investments to ensure the data they contain is reliable, accurate and complete and remains so over time.
2
Quality control routine
To steadily build confidence and trust in data, it is important to set down good habits and build these into everyday processes. By putting the right data hygiene practices into place, companies can avoid the high costs and delays caused by data remediation exercises, which can run into millions of dollars or euros. Spending just a fraction of that amount on embedding good practice and dedicated resources is cost effective and will pay dividends in the long term.
Operationalising data quality standards is important. These include naming conventions and data standards, data links with related content and data completeness guidelines. These need to be applied consistently on a global basis.
Not all data quality errors are equal, so it is important to be able to flag serious issues for urgent action and tracking of error origins, so additional training or support can be provided.
3
Alignment with recognition and rewards systems
Recognition, via transparency, will continue to inspire good performance, accelerate improvements and bed in best practice, which can be readily replicated across the global organisation to achieve a state of continuous learning and improvement.
Knowing what good looks like, and establishing KPIs that can be measured against, are important too. Where people have had responsibility for data quality assigned to them as part of their roles and remits, it follows they should be measured for their performance, with reviews forming part of job appraisals, and rewarded for visible improvements.
4
Creating a mature and disciplined continuous improvement programme
Gens & Associates’ 2018 research found that life sciences companies with a Regulatory ‘continuous improvement programme’ (CIP) have 15% higher data confidence levels, 17% are more likely to have achieved real-time information reporting, and 21% have higher efficiency ratings for key RIM capabilities.
Continuous improvement is both an organisational process and a mind-set. It requires progress to be clearly measured and outcomes tied to business benefits. A successful CIP in Regulatory data management combines anecdotal evidence of the value that can be achieved and clear KPIs (cycle time, quality, volume etc.) that teams can aim towards and be measured against.
At its core, continuous improvement is a learning process that requires experimentation with ’incremental’ improvements. Establishing good governance and measuring for and reporting on improvements and net gains and how these were achieved (what resources were allocated, what changes were made, and what impact this has had), will be important too.
5
Data standards management
Today in many life sciences companies, data is not aligned across the organisations and standards vary or simply do not exist. Ask people in regulatory, pharmacovigilance, supply chain, and quality how they define a ‘product’ or how many products their company has, and answers will probably vary.
The more that all companies keep to the same regimes and rules, the easier it will become to trust data, and what it says about companies and their products - as it becomes easier to view, compare, interrogate and understand who is doing what, and how, at a community level.
Evolving international standards such as ISO IDMP and SPOR mean that companies face having to add and change the data they are capturing over time. To stay ahead of the curve, life sciences companies needs a sustainable way to keep track of and adapt to what’s coming.
Delegating monitoring activity to persons responsible for quality is unrealistic, as there is so much detail to keep track of. Meanwhile regulatory specialists may understand the broad spectrum of needs, yet not how to optimise data preparation for the wider benefit of the business. It may be worth seeking external help here, to attain an optimal balance between regulatory duty and strategic ambition.
Long-term AI potential depends on data quality sustainability investment today
The important takeaway from all of this is companies cannot confidently innovate with AI and process automation based on data that is not properly governed.
With emerging technology’s potential advancing all the time, it is incumbent on organisations to formalise their data quality governance and improve their ongoing data hygiene practices now, so they are ready to capitalise on AI in meaningful ways in the near future.
About the authorS
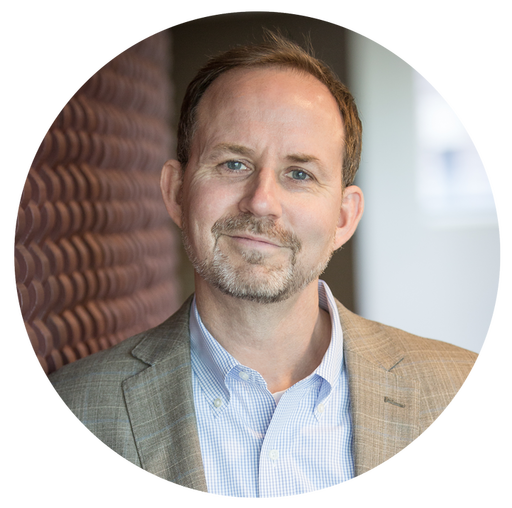
Steve Gens is the managing partner of Gens & Associates, a life sciences consulting firm specialising in strategic planning, RIM programme development, industry benchmarking, and organisational performance.
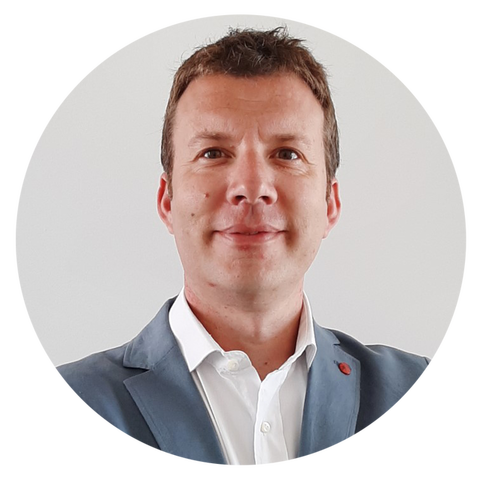
Remco Munnik is associate director at Iperion Life Sciences Consultancy, a globally operating company which is paving the way to digital healthcare, by supporting standardisation and ensuring the right technology, systems and processes are in place to enable insightful business decision-making and innovation.
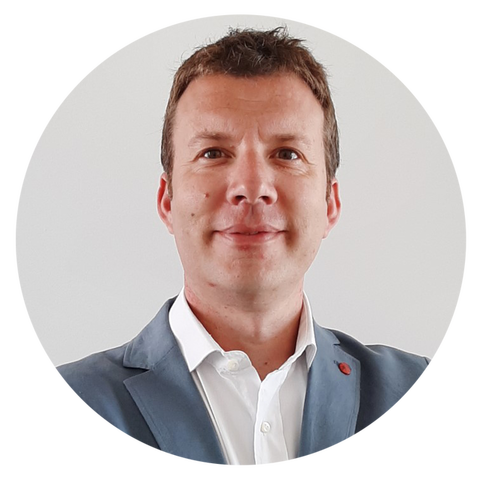