AI is an essential technology for healthcare companies. In the pharma and medical devices industry, AI rapidly accelerates drug discovery and manufacturing and enables supply chain efficiencies. For healthcare payors and providers, AI tackles staff and skill shortages and increases administrative efficiency.
Although AI capabilities are still in their early stages, it has already begun revolutionizing the drug discovery process by streamlining research and potentially reducing the time and cost required to bring new drugs to market, addressing a broad range of unmet needs. AI also shows great promise in medical diagnostics, enhancing surgical precision, speeding up image analysis, and improving disease diagnosis.
Automation of routine tasks by AI can allow healthcare providers to see more patients, while personalized care, predictive analytics, and faster diagnoses can lead to better patient outcomes and a more positive patient experience.
Developing accurate AI models requires access to extensive, high-quality patient data from diverse populations. The use of healthcare data for AI development raises significant privacy concerns for both organizations and individuals. For instance, employing large language models (LLMs) like ChatGPT to improve access to health information could impact patient privacy. Therefore, developing new data-sharing regulations and methods that preserve privacy is essential for the widespread adoption of AI applications in healthcare.
How pharma companies should approach AI investmen
The matrix below details the areas of AI in which pharma companies should focus their time and resources. They should invest in the technologies shaded green, explore the prospect of investing in the technologies shaded yellow, and ignore the technologies shaded red.
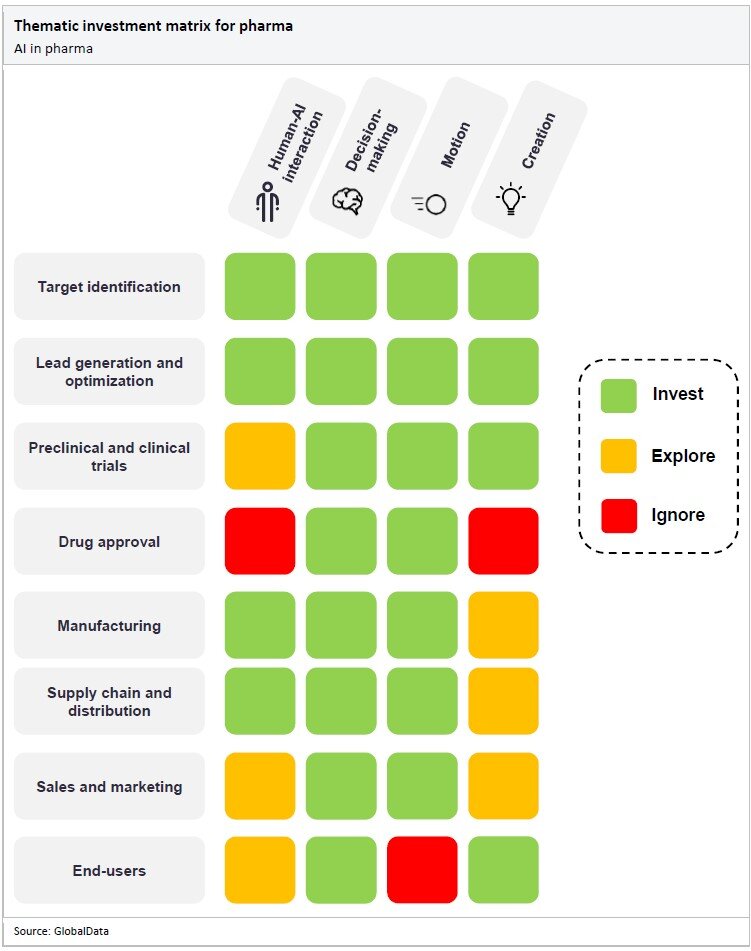
Although AI is beneficial for areas across the pharma industry, companies should focus on investing in AI for drug discovery, accelerating processes, and enhancing efficiency in manufacturing and supply chains.
Advancements in AI have significant potential to improve the quality of care and health outcomes. This includes increasing the frequency and speed of getting new drugs to market, thereby increasing profitability for pharma companies. AI also enables faster and earlier diagnoses, access to improved clinical trials, and the potential for personalized medicine.
In drug discovery, AI can analyze vast amounts of data to detect patterns and trends and assess the biological performance of drug candidates to find the best for clinical testing. GenAI, for example, can predict novel drug candidates or molecular structures based on patterns and trends found in data.
Biotechnology company Insilico Medicine announced in April 2023 the successful discovery of a CDK8 inhibitor for the treatment of cancer using its GenAI-driven learning platform named Chemistry42. In February 2024, 4B Technologies’ drug FB1006, a new potential treatment for amyotrophic lateral sclerosis (ALS), entered into an investigator-initiated clinical trial. The drug was discovered and developed entirely using AI, from target identification to efficacy assessment. AI also enabled researchers to speed up patient screening.
Generative AI can also be used to design novel chemical compounds. For example, researchers at the University of Toronto have developed an AI system that can create proteins not found in nature (Lee et al., 2023). The system uses the same technology as OpenAI's Dall-E image creation platform. In the future, this could help discover new drug candidates with unique properties, potentially creating treatments for diseases that currently have no effective options.
AI can also accelerate and digitalize clinical trial processes so studies can be completed faster. AI can optimize the design of patient-centric clinical trials and allows technologies such as wearables, heart monitors, and body sensors to collect patient data remotely and less invasively. AI algorithms specifically help data collection by identifying patterns and improving the accuracy of datasets. Remote monitoring and decentralized clinical trials provide an attractive way to increase patient participation and access more diverse groups of participants.
Pharma companies should invest in AI tools for efficiency in manufacturing, distribution, delivery, and streamlined supply chains. Through AI, many areas of a medical supply chain can be automated, including inventory tracking, and managing and restocking processes. Investing in AI-powered tools such as robotics and drones will also speed manufacturing processes by assisting in supply delivery. ChatGPT could create optimization plans and predictive maintenance schedules and detect anomalies in available information.
How AI helps tackle the challenge of drug discovery and development
Drug discovery and development is extremely expensive and time-consuming. It takes between 12 and 18 years for a drug to reach the market, at an average cost of around $2.6 billion. Drug discovery processes alone involve many stages, including target identification and validation, assay development and screening, hit identification, lead optimization, and selection of candidates for further clinical development. A successful outcome is also unlikely, with only 10% of drug candidates making it through clinical development (Sun et al., 2022). The low success rate and high cost have created a need for alternate approaches.
Over the past several decades, advances in computational technology have allowed increased exploration of the vast chemical space. Computer-aided design and drafting, called “in silico” approaches (experimentation performed by a computer), are widely used to enhance traditional drug discovery methods and can reduce the time and cost of drug development, with significantly higher hit rates. However, the success rates are still low.
AI has shown enormous potential to further enhance in silico methods by rapidly understanding and exploring the expanding chemical space, driven by the ever-growing amount of big biomedical data. Training AI on the vast amounts of genomic data, health records, medical imaging, and other patient information has allowed an increased understanding of the biological mechanisms of diseases. AI-assisted mapping of disease pathways and protein and drug interactions through specialist vendors has created a deeper understanding of targets and has assisted in identifying novel proteins and genes that can be targeted to counteract them.
AI can also be used to predict 3D structures of targets and accelerate the design of appropriate drugs that bind to them, through protein structure databases such as DeepMind’s AlphaFold. Once a set of promising lead drug compounds has been identified, AI can then assist in candidate drug prioritization, ranking molecules for further assessment.
Aside from identifying drug targets, AI has transformed the virtual screening of compounds, such as identifying those that can bind to “undruggable” targets, de novo drug design, drug repurposing, and identification of treatment response biomarkers (Abou Hajal et al., 2024). AI algorithms have shown the potential to condense a typical four- or five-year exploratory research phase into less than a year, significantly reducing the time and cost to get a drug to market, which is particularly important for indications with few treatment options.
There have been some significant milestones in the use of AI in drug discovery since 2023. In January 2023, Absci became the first company to create and validate de novo antibodies in silico using zero-shot generative AI. Creating de novo therapeutic antibodies in silico could reduce the time it takes to get new drug leads into clinical trials by more than half. The zero-shot generative AI model generates novel antibody designs that are not found in any existing databases.
In February 2023, Insilico Medicine was granted orphan drug designation by the FDA for INS018_055, a small molecule inhibitor treatment for idiopathic pulmonary fibrosis (IPF). INS018_055 was discovered and designed through the company's generative AI platform, Pharma.AI. That year, the drug was the first of its kind to be given to humans in a global, randomized Phase II clinical trial. The total time from discovery initiation to the start of Phase I took under 30 months for INS018_055, representing a significant increase in speed for therapeutic asset development in the pharma industry.
In May 2024, Google DeepMind released the third version of AlphaFold. The previous models were built to solve the issue of predicting the 3D structure of a protein from its amino acid structure. AlphaFold 3 does more by predicting the structure of almost all biological molecules and modeling the interactions between different molecules. This marks the first time a single system can predict interactions between almost all molecules with this much accuracy. How a molecule and biological systems will react depends on the interactions between different molecules. Usually, it takes years for scientists to understand molecular interactions; with AlphaFold 3, this will take significantly less time.
Despite these advancements, most novel drugs developed using AI are in the preclinical or discovery stages, and it could be many years before an AI-based therapy is approved. While AI has shown the potential to transform drug discovery processes, it faces many challenges. These include the quality and appropriateness of data, continued assurance of drug safety and efficacy, educating the scientific community to increase buy-in, and issues around intellectual property rights.
Although the impact of AI on traditional drug discovery is in its early stages, we have already seen that when layered into a traditional process, AI-enabled capabilities can substantially speed up and reduce the costs of running expensive experiments, which has the potential to be transformative for patients, medical providers, and the pharma sector.
How AI helps tackle the challenge of low success rates of clinical trials
Clinical trials face many challenges, including complexity, inflated costs, regulations, patient access, and recruitment. Patient recruitment is essential for the success of a clinical trial. However, around 80% of clinical trials globally fail to recruit and retain enough patients, leading to trial delays and excessive costs (Desai, 2020). Clinical trials are becoming increasingly complex, further intensifying the challenges in executing them. These issues can potentially limit the development of innovative drugs.
Clinical trials are essential to ensure the safety and efficacy of all potential drugs before they enter the market. However, this process remains slow, expensive, and unpredictable. AI tools can potentially improve the success and efficiency of clinical research.
The impact of poor recruitment on a trial is immense in terms of time and associated financial burdens. AI can identify potential candidates for clinical trials by analyzing vast amounts of patient data and medical records. It can also analyze social media content to identify specific regions where a condition is more prevalent, narrowing the search for an optimal cohort. Once the desired patient subgroups are detected, AI can also assist and accelerate trial recruitment.
Traditionally, eligible patients are found though hospitals or clinics, but often, when recruiting large numbers of people, only a few are eligible. AI technologies can alert medical staff and patients to trial opportunities and reduce unnecessary patient screening. It can also help simplify entry criteria to make it more accessible for potential participants. AI-based analysis can also provide insight into participant behavior, and researchers can use this information to design more efficient and effective clinical trials.
Furthermore, AI can analyze patients’ genetic makeup, other physiological data, and lifestyle factors, helping to identify specific patient populations likely to benefit from a particular drug. A personalized treatment plan for a potential high-risk cancer patient could involve earlier screening, which may help with earlier diagnosis and treatment and lead to improved quality of life for the patient.
One example is Deep 6 AI, a platform that uses AI to streamline and increase patient recruitment for trials. The platform analyses large amounts of medical data, such as electronic health records (EHRs), pathology reports, and clinical notes, to find candidates that meet the criteria for a trial.
The average dropout rate for traditional clinical trials is about 30%, depending on the trial phase (CenterWatch, 2016). Patients may discontinue trial participation for various reasons, including inconvenience, the number of visits required, complex trial protocols, lack of support, and little or no reimbursement of expenses. Through AI, health assessments can be performed remotely in patients’ homes, improving patient experience and ease during clinical trials and boosting patient participation and retention.
Decentralized clinical trials reduce reliance on in-person trial sites and overcome any barriers related to inaccessibility, especially for underrepresented populations. Patients' vital signs and other information can be collected remotely through wearable devices and mobile apps. Real-time tracking of medication can also help reduce medical non-adherence. The traditional process of manually tracking medication, through reliance on a patient’s memory, is prone to error and can limit clinical trial outcomes.
One example is the clinical trial management platform AiCure, an interactive medical assistant that helps to spot patients at risk of non-adherence. Patients in a clinical trial sign up to the platform via a mobile app, where they record their progress and receive reminders to take medication. Patients video themselves taking their clinical trial medication as proof, and the AI platform can then identify the correct patient and pill to ensure the patient is engaging with the clinical trial properly.
Each clinical trial generates vast amounts of data that researchers must manually review to uncover meaningful insights. AI can analyze this data efficiently, finding patterns that may be missed by human analysis. For example, AI-based models can predict the toxicity of a potential drug candidate, helping researchers select suitable compounds that can then be used in a trial. Organizations can also use AI technologies to find suitable existing data for new trials, reducing the need to start from scratch across trials and speeding up the design process.
While the application of AI shows promise to increase the efficiency of clinical trials, universal data management and inherent bias in data need to be addressed to use AI successfully. The differences in regulation between countries limit the scope of collaboration and cooperative research and may restrict the ability to train AI systems at a global scale. AI-based systems also raise data security and privacy concerns. Therefore, maintaining the confidentiality of medical records used in clinical trials is crucial.
How AI helps tackle the challenge of precision and personalized medicine
There has been an important shift to precision and personalized medicine in recent years. The classic one-size-fits-all method is changing to tailored treatments that consider individual differences in genetics, lifestyle, and environment. This shift acknowledges that even if patients share the same diagnosis, their unique characteristics can lead to different responses to the same treatment, affecting treatment outcomes. AI is an important tool for overcoming these challenges and supporting the development of personalized care.
One key obstacle to personalized healthcare is the management and analysis of complex and diverse medical data (e.g., medical history, reports, activity data from wearable devices, and real-time monitoring). The healthcare and pharmaceutical industries generate abundant data from many sources, including physician notes, pathology reports, EHRs, patient registries, genomics, clinical trials, wearable devices, and many more.
A human cannot efficiently process and analyze all this data to create a personalized treatment plan for each patient. AI, in contrast, can process all the medical data, recognize patterns, and provide insights from these datasets. For example, machine learning (ML) algorithms can be used to identify relations between a mix of tests, medical history, and allergy information to propose a tailored treatment plan.
AI can also significantly advance the development of precision and personalized medicine by identifying novel predictive biomarkers critical to tailoring specific treatments to individual patients. For example, Ocean Genomics’ AI platform identifies predictive variants in messenger RNA (mRNA) regarding a patient’s expected response to a drug, and the technology is then used in drug discovery by pharmaceutical companies to develop personalized treatments.
Similarly, platforms like Paige AI focus on identifying predictive and prognostic biomarkers to assist in connecting patients with the correct treatment, while Certis Oncology Solutions’ CertisAI predictive medicine platform, launched in April 2023, uses data analytics and ML to study predictive biomarkers, enhance treatment strategies, and improve drug success rates.
The effectiveness of AI heavily relies on integrating big data, as big data is essential for the development and application of AI in the precision and personalized medicine field. AI models rely on high-quality data to enhance their decision-making capabilities. As these models process and train on more data, their accuracy and efficiency improve, leading to actionable insights that enhance drug development and precision in treatment and reduce the time and cost of bringing new drugs to the market.
GlobalData, the leading provider of industry intelligence, provided the underlying data, research, and analysis used to produce this article.
GlobalData’s Thematic Intelligence uses proprietary data, research, and analysis to provide a forward-looking perspective on the key themes that will shape the future of the world’s largest industries and the organisations within them.