Company Insight
Sponsored by Avenga
LLMs for pharma and bioscience, applications and challenges
Jacek Chmiel, Avenga’s IT Market Expert, lets you in on what's next for rapid LLMs adoption in the pharma sector.
Main image: Large Languages Models are no longer a novelty in the pharma and bioscience sectors
Generative AI became a household name in 2023, and the vast majority of enterprises in the world have already completed their first experiments with large language models.
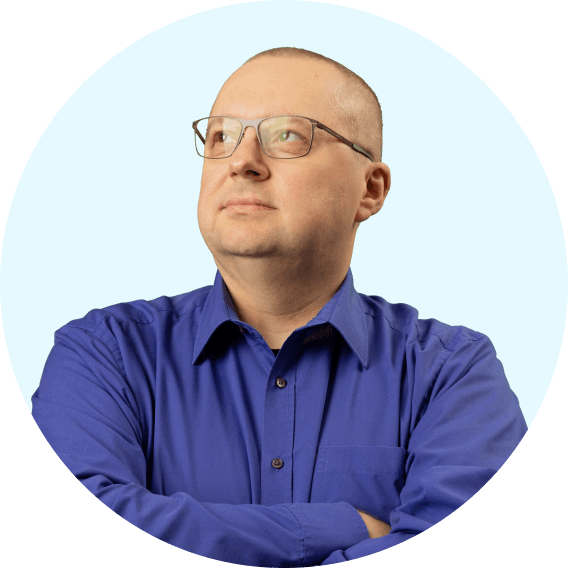
Jacek Chmiel, IT Market Expert
And that includes pharmaceutical companies as well. The honeymoon period for LLMs is over. Everybody has a chatbot or two, the question is what to do next. New products are available on the market and there are more options in terms of new LLM models and services, the pace of innovation is accelerating.
Now the question is where is the actual business value, where does new AI lead to more operational efficiency, lower risks, and improved business outcomes? I’m going to focus on short-term, available solutions with the highest potential of a positive return of investment.
Practical applications
Large language models are great with large amounts of textual data. And there’s a lot of untapped potential in being able to work with this data in a much more efficient way than before.
One of the greatest hopes is to be able to process vast amounts of scientific literature, to be able to extract valuable insights, and (finally) turn text data into information. Plus, it’s much easier to talk to a bot instead of attempting to use manual search for keywords. Modern large language models are great tools to not only extract value out of tons of data but also to generate new hypotheses and propose entirely new ideas. They can also help to design more targeted and efficient clinical trials.
Electronic Health Records (EHR) are (on the surface) fully digitalized, technically electronic, and queryable using various technologies, but there’s still an ocean of unstructured text. Even though we had Natural Language Processing technologies for years, the outcomes were often disappointing, now it’s time to give those efforts another shot using orders of magnitude better models and techniques (such as Retrieval Augmented Generation).
The virtual patient advisor is another interesting application of LLMs. Doctors are busy, their time is expensive, and they can be overwhelmed and not empathetic enough as a result. Patients get 24/7 access to personalized, empathetic, and well-educated bots to help them from a source they can trust instead of going to random internet forums for a piece of information and advice.
Modern, multi-modal models persistently scan social media for videos, pictures, and posts to analyze customer sentiments about specific products (drugs, services), to better respond with improved marketing content and strategies.
The generative power of large language models is already well-known for all the software developers who are using LLMs as their everyday coding assistants. That’s because those models are good with any grammar and language (human or artificial) that can be expressed as tokens, and that includes molecules down to the level of single atoms. Models predict the next atom in the sequence or a functional group of molecules given specific properties of the molecule as the target of their optimization.
Generative AI is already used to analyze drug interactions and help with drug repurposing.

Adoption barriers and challenges
Humans interacting with generative AI tend to take accuracy for granted, it’s the computer after all and it’s supposed to be accurate for decades, hasn’t it? This is what we have to unlearn when working with generative AI. Because of the surrounding hype about actual efficiency gains, it takes time and effort to verify the results and accept ‘reduced’ efficiency gains to verify the results. The initial impressions of human-like responses cannot blind us. In the case of pharma researchers, scientists, and medical doctors, they have to be more vigilant and skeptical than regular users looking for trip advice, the consequences are more serious, legal, and ethical. Expect a significant efficiency boost of tens of percent (instead of advertised thousands), which still is a great achievement of modern language models.
Hallucinations of the models can often be reduced to a very low percentage (using RAGs, models with huge token windows), but it requires a lot of skills and experience to use those techniques.
There’s a fundamental strategic dilemma that directly impacts business outcomes. And that includes the choice between cloud services for LLMs (GPT-4, Gemini) and locally tuned open source models (LLama2, Mistral) which is a tradeoff between time to market, operational cost, data privacy, and response latency. There are strong proponents of large open-source language models because of the sensitivity of the data and avoiding the creation of strong vendor lock on particular cloud services, given their lifespans are not guaranteed in such a hot innovative environment.
The cost per transaction can be an unforeseen pain when not estimated properly, chatting to one-page PDFs using a chatbot is probably not the best use of computational resources and money. Also, fine-tuned prompts and model tunings are not guaranteed to work with other models and even with the newer versions of existing models, and that reduces ROI.
Reproducibility requirements, evidence-based processes, and validation, which are so important in bioscience, are not well addressed because of not up-to-par explainability of large language models. XAI for LLMs is a work in progress, lagging behind the actual innovation focused on building even larger and smarter models.
Data availability and data quality problems are not going anywhere. Yes, the LLMs can be tuned to provide answers, correct on the surface, but their quality is not going to magically overcome the limitations of actual data that is fed to them.
Conclusions
With the great power of generative AI and large language models comes a great responsibility of its users, on an individual level and organizational level.
The safest strategy is to use LLMs where the benefits are visible, taking into account the validation of the answers, and the IT cost of evolving and maintaining the technology.
Keeping a hand on the pulse of technology in a business-oriented Proof of Concept stream is also a very good idea to focus only on what brings value today, making sure there’s no missed opportunity related to the latest and greatest in generative AI.
Would you like to have a more personalized conversation about how LLMs can bring value to your organization? Get in touch!